Google Shopping is a Google Ads product that allows advertisers to serve feed-based ads on the search engine results page (SERPs).
The auction for Shopping Ads works in a similar way to Google Text Ads, in the sense that the auction is query-based.
However, Google Shopping does not target keywords and uses the feed (and a few other factors) to determine when and where to serve ads.
Here’s an example of the Google Shopping results on a SERP:
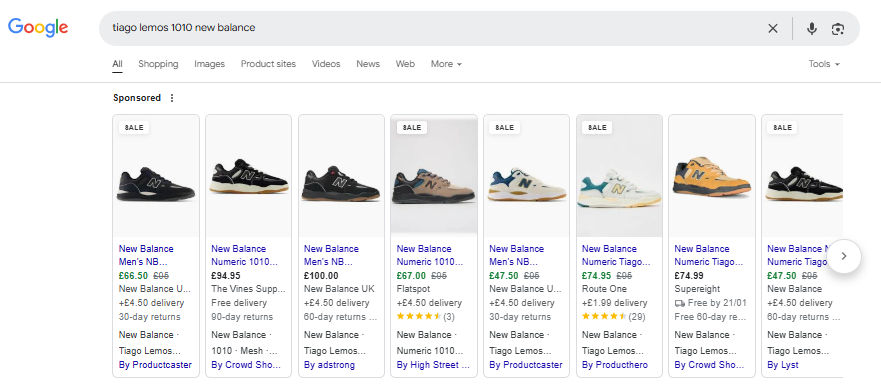
Advertisers are set to ramp up their spending on U.S. retail media search ads, with a projected 23.4% year-over-year growth in 2028, pushing the total spend to $76.83 billion.
Google Shopping offers advertisers the freedom to serve:
- Product images.
- Clear product titles.
- Content-rich descriptions.
- Upfront pricing.
- Promotions.
- Shipping costs.
Google Shopping allows advertisers to inform searchers about their products prior to clicking through – and when compared to standard text ads – has the potential to drive better-qualified traffic.
From multinational retailers to local bakeries, hundreds of thousands of brands use Google Shopping to get their products in front of searchers every day.
How To Find Success With Google Shopping Ads?
Many factors determine how online advertising performs, from key performance indicators (KPIs) to pricing, payment options, imagery, site speed, the social responsibility of a company, and more.
However, looking solely from an ad platform perspective at Google Shopping, the one factor that will determine success is data.
- Product Feed: The data within your feed should be high quality, accurate, and well-planned. This is the heart of Google Shopping and is a huge factor in determining the search queries your shopping ads will enter the auction for. Where possible, ingest additional data that will help feed bidding strategies, reports, and more with valuable insights about your products.
- Segmentation: There are many ways to segment Google Shopping campaigns: by margin, product categories, search query length, best sellers, and more. Segmentation and structure are important because this is where advertisers can control their budgets, set targets, and lay the foundations for scaling spend.
- Budgets and Bidding: If your structure and segmentation lend themselves to your KPIs, you’ll be able to set budgets with confidence and build a portfolio of bidding targets that will work towards the correct goal.
- Refinement: There aren’t any keywords, but there are negative keywords. Use these to refine your campaigns and ad groups to enter auctions for search queries that align with your KPIs. It may be that for upper funnel generic queries, you want to serve a certain category but not another; this is a perfect use case for negating queries and funneling traffic.
- Performance Max: I couldn’t talk about shopping without mentioning PMax. All of the above applies; the only difference is that segmentation works slightly differently with asset groups and one single target, which is set at the campaign level vs. ad group level for Google Shopping.
With these basics in place, from the moment you activate your campaigns, you’ll be gathering data and learning.
This learning is the backbone of shopping campaigns, providing Google (and the bidding algorithm) crucial data all the way down to an SKU level.
Over time, you’ll start to uncover a wealth of insights, such as:
- Which products have the highest conversion rate?
- How does engagement look for category A when served for upper funnel search queries?
- What happens to the conversion rate when products A, B, and C drop out of stock?
This data feeds machine learning as Google understands how your products perform across hundreds of thousands of touchpoints.
This model fits most ecommerce brands with multiple stocks of each item to gather learnings overtime on what works and what doesn’t.
But if you’ve only got one of every product, how can you drive success on Google Shopping when once a product’s gone, it’s gone?
What Business Models Have One Of Each Product?
- Auctions, e.g., eBay.
- Marketplaces, e.g., Etsy.
- Second-hand/pre-loved, e.g., Vinted.
- A mix of the above. Typical retailers who have adopted a marketplace feature or a pre-loved arm of their business, such as Farfetch.
The scale of the business, vertical, market, etc., all play a role in determining the stock of each SKU.
Take a brand like eBay, a global online marketplace with both auction and “buy it now” functionality. They have thousands of items where the stock level is above one, and thousands where it is one of one.
There are thousands of auction houses, second-hand retailers, marketplaces, and more that have a similar setup, but on a smaller scale.
But for this post, we are focussing solely on one of one product.
How Does This Business Model Impact Google Shopping?
This campaign type thrives on data, and this flows through every layer, from the bidding strategy down to individual SKU performance.
The feed is the heart of Google Shopping, and with the SKUs changing frequently (depending on the business), accruing data on which SKU performs the best/worst works differently as SKUs sell through and may not be in the feed again for weeks, months, or in some cases, ever again.
There are a number of considerations that need to be taken into account:
- Learning: With only one of each SKU, items may sell out quickly, whereas some items may be in the feed for longer. Bidding algorithms will struggle to gather data to optimize toward your KPIs, and a lack of historical data will be limiting for machine learning, especially at a product level.
- Feed: The data within your product feed should be rich, up-to-date, and aligned with your paid media goals. This is even more important when SKUs are being added/removed frequently, as this will cause instability with learning, crawls, and more.
- Reporting: With one-of-a-kind SKUs, the interpretation of the data within the ad platform is critical; it’s not like you can filter by sales > 0 over a date range and decide how to structure your campaigns, as many SKUs will have been and gone.
- Automation: Bid strategies can certainly be used, but unlike traditional retailers who may have in-platform ROAS/CPA targets that remain fairly stable, the intricacies of category performance and knowing exactly what products have sold is critical as this is ever-changing and will impact how you feed data into machine learning.
- Budget Allocation: When building for the long term, fluctuations in performance make it difficult to set budgets to get the most out of your media spend. Watertight reporting is essential, and communication between teams is key to helping spot trends, plan inventory ahead of time, and stay as efficient as possible.
- Dynamic Retargeting (and PMax): Dynamic retargeting uses the feed to serve product ads to audiences (e.g., website visitors who have added an item to a cart and not purchased) and can be run in isolation or as part of PMax. Having one of every product creates a disconnect as multiple users could be interested in one item, and when it’s sold, it’s gone from the feed.
These are just a snapshot of the limitations, and there are more.
But that certainly doesn’t mean it’s a non-starter.
A different approach is needed, compared to Google Shopping, for a traditional e-commerce model. Above all, communication and planning will be the backbone for success as these campaigns most certainly don’t fall into “set and forget” paid search management.
Can You Scale Google Shopping For This Business Model?
Absolutely.
This will require a fresh perspective on how you report, optimize, and plan your media budget, but it’s certainly achievable.
Look at eBay. It spends >$150 million each year on Google Ads, with the majority being through Product Listing Ads (PLAs).
Here are a few approaches that are tried and tested:
Reporting
Product-level reports are going to be useful for any ecommerce business. However, with products dropping in and out of stock frequently, a focus on categories (or bespoke groupings) is essential.
Say you’re a home furniture auction house with a large inventory. In the mass of data, you’ll need to find trends, and these trends sit within various categories, which are formed from aggregated product data over time.
This could be:
- Top-searched designers or brands.
- Most purchased colors of category A.
- Share of search by category across AOV brackets.
This data will feed into almost all strategies and tactics adopted in the account, from structuring to forecasting and setting bidding targets.
This reporting can be automated and then queried to provide each stakeholder with a different view of performance that all leads back to driving growth through Google Shopping:
- Buyers may want to see which categories or designers are indexing highly by search volume to feed into planning, which, in turn, helps Google Shopping as the products/categories that are performing the best are then stocked moving forward.
- Paid search teams will want a view of how ROAS/CAC has trended over time by category to know how to set realistic targets at the campaign, ad group, and product group levels.
- Analytics teams need a view of the time lag between the first session date by campaign and the purchase date to provide feedback to marketing teams on how to accurately report on Google Ads performance.
Optimization
Google is going to struggle to gain enough data to optimize at a product level.
Mirroring your reporting, you will need a view of performance at the category (or another grouping) level, as individual product performance isn’t going to feed into your campaigns as it would for a typical ecommerce store sat on the stock.
You’ll need to do the work analysing performance across multiple segments to build a picture of how each category performs to then set budgets and bidding targets and maintain the day-to-day tasks required to manage Google Shopping campaigns.
Product Feed
It is critical that your feed is optimized and you are ingesting as much supplemental data as possible (within reason).
This data will feed into your Google Shopping campaigns, and the time invested will pay for itself down the line.
Take the furniture store example. It can supplement its data with era, designer, etc. When new items are added, this additional data can help group products into segments with realistic targets and budgets vs. being dropped into a top-level category and leaning on product performance to determine what SKUs to serve.
Above all, there has to be ownership and a process for adding SKUs to the feed.
Although products will be moving in and out of your feed frequently, there will likely be cohorts of SKUs that will remain in the feed for a while, which you should keep an eye on as these may need removing/scaling back in line with efficiency.
Summary: Advertisers Will Need To Think On Their Feet
A great deal of the work involved in navigating this business model and scaling Google Shopping happens outside of the ad accounts.
Advertisers need to interpret and share data across the wider business, and this process works both ways.
What are buyers in the company looking at bringing in and where would this sit with the Google Shopping strategy? Are there categories trending upwards that can be shared with the wider team to capitalize on?
Without stable product data, advertisers will need to think on their feet and get fully ingrained within the business, which in 2025 is essential – whatever the business model.
More Resources:
Featured Image: BestForBest/Shutterstock